Segmentation of Brain Tumor Analysis of Fuzzy C-Means and K-Means
DOI:
https://doi.org/10.31033/abjar.2.3.5Keywords:
brain tumor, fizzy, c-means, k-meansAbstract
Currently, the different algorithms for detecting tumor range and shape in brain MR images are being implemented and it is now possible to find out the degree of tumor with regard to the given tumor area. The information was gathered via research of various statistical analysis methods which are all based on those individuals who have been diagnosed with brain tumors, and then risk factors and symptoms that appear for all individuals diagnosed with brain tumors were discovered. The advancement of research in medicine day and night aims to provide modern therapeutic approaches. The surgeon physically examines this image in order to identify and diagnose brain tumors. However, this procedure accurately measures the stage and scale of the tumor and accurately distinguishes the stage of the tumor based on the location of the tumor. This dissertation employs k-means and fuzzy c-means algorithms to segment brain tumors and classify tumor cells using CNN (convolution neural network). This approach enables the accurate and reproducible segmentation of tumor tissue equal to manual segmentation. Additionally, it decreases research time and accurately determines the stage of tumor from a given region of tumor.
Downloads
References
Samir Kumar Bandhyopadhyay, & Tuhin Utsab Paul. (2013). Automatic segmentation of brain tumor from multiple images of brain MRI. International Journal of Application or Innovation in Engineering & Management (IJAIEM), 2(1).
A. Meena. Spatial fuzzy c-means PET image segmentation of neurodegenerative disorder. Indian Journal of Computer Science and Engineering (IJCSE).
Suman Tatirajua, & Avi Mehta. (2008). Image segmentation using k-means clustering em and normalized cuts. IEEE Trans. Parallel Diatribe. Syst., 19(5), 710–720.
Ajala Funmilola A, Oke O.A, Adedeji T.O, Alade O.M, & Adewusi E.A. (2012). Fuzzy k-c-means clustering algorithm for medical image segmentation. Journal of Information Engineering and Applications, 2(6).
Beshiba Wilson, Julia Punitha, & Malar Dhas. (2014). An experimental analysis of fuzzy c-means and k-means segmentation algorithm for iron detection in brain swi using matlab. International Journal of Computer Applications 104(15).
Samarjit Das. 92013). Pattern recognition using the fuzzy c-means technique. International Journal of Energy, Information and Communications, 4(1).
Krishna Kant Singh & Akansha Singh. (2010). A study of image segmentation algorithms for different types of images. International Journal of Computer Science Issues, 7(5).
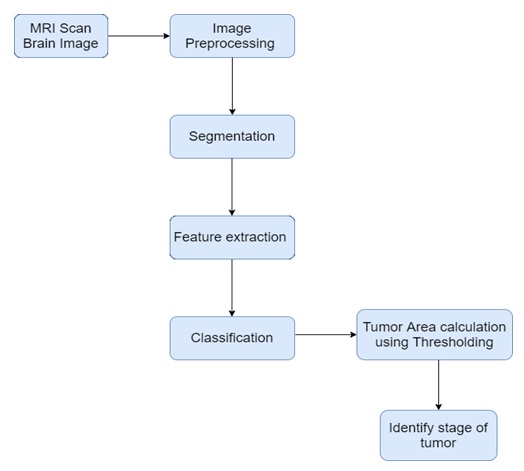
Downloads
Published
How to Cite
Issue
Section
License
Copyright (c) 2023 Vilas Karanje, Dr. Ashitosh Gaikwad

This work is licensed under a Creative Commons Attribution 4.0 International License.