Effects of Self-Driven Reinforcement Learning
Keywords:
reinforcement learning, structure, limitations, agentsAbstract
Structured Learning, unstructured Learning, and reinforcement Learning is the three main components of machine Learning (ML). In this paper, we'll focus on reinforcement Learning, which is the final stage. There are numerous methods of reinforcement learning, and we'll go over some of the more popular ones. Software agents that use reinforcement learning to maximize their rewards in a given environment are known as reinforcement agents. Extrinsic and intrinsic rewards are the two main classifications of rewards. It's a specific outcome we get after following a set of rules and accomplishing a specific goal. Rather than monetary gain, a better example of an intrinsic reward is the agent's eagerness to learn newly acquired expertise that may prove beneficial in the future.
Downloads
References
Ng, A. Y., & Russell, S. J. (2000). Algorithms for inverse reinforcement learning. Proceedings of the Seventeenth International Conference on Machine Learning, pp. 663–670.
Karpathy, & M. Van De Panne. (2012). Curriculum learning for motor skills.
Barto, A. G. (2013). Intrinsic motivation and reinforcement learning. Intrinsically Motivated Learning in Natural and Artificial Systems, Berlin, Heidelberg: Springer, pp. 17–47.
Wilson, A. Fern, & P. Tadepalli. (2014). Using trajectory data to improve bayesian optimization for reinforcement learning. J. Mach. Learn. Res.
N. Bougie, & R. Ichise. (2020). Skill-based curiosity for intrinsically motivated reinforcement learning. Mach. Learn.
T. D. Kulkarni, K. R. Narasimhan, A. Saeedi, & J. B. Tenenbaum. (2016). Hierarchical deep reinforcement learning: Integrating temporal abstraction and intrinsic motivation.
Abhay Singh Hyanki, Shweta Meena, & Tarun Kumar. (2021). A survey on intrinsically motivated reinforcement learning. International Journal of Engineering Research & Technology, 10(5), 1150-1153.
Kulkarni, Tejas D., Narasimhan, Karthik R., Saeedi, Ardavan, & Tenenbaum, Joshua B. (2016). Hierarchical deep reinforcement learning: integrating temporal abstraction and intrinsic motivation. Proceedings of the 30th International Conference on Neural Information Processing Systems.
R. Salakhutdinov, & A. Mnih. (2008). Bayesian probabilistic matrix factorization using markov chain Monte Carlo.
Raffin, S. Höfer, R. Jonschkowski, O. Brock, & F. Stulp. (2017). Unsupervised learning of state representations for multiple tasks. ICLR.
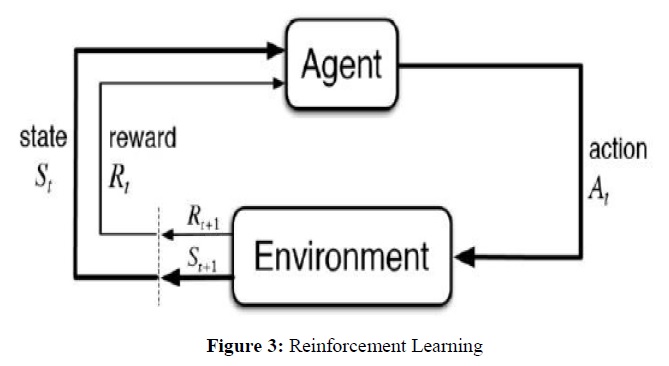
Downloads
Published
How to Cite
Issue
Section
ARK
License
Copyright (c) 2022 Sanjay Rao, Rohit Bharat

This work is licensed under a Creative Commons Attribution 4.0 International License.
Research Articles in 'Applied Science and Biotechnology Journal for Advanced Research' are Open Access articles published under the Creative Commons CC BY License Creative Commons Attribution 4.0 International License http://creativecommons.org/licenses/by/4.0/. This license allows you to share – copy and redistribute the material in any medium or format. Adapt – remix, transform, and build upon the material for any purpose, even commercially.