Trajectory Data to Improve Unsupervised Learning and Intrinsic
DOI:
https://doi.org/10.5281/zenodo.10656240Keywords:
trajectory data, learning, motivationAbstract
The three primary components of machine learning (ML) are reinforcement learning, unstructured learning, and structured learning. The last level, reinforcement learning, will be the main topic of this study. We'll cover a few of the more well-liked reinforcement learning techniques, though there are many more. Reinforcement agents are software agents that make use of reinforcement learning to optimize their rewards within a specific context. The two primary categories of rewards are extrinsic and intrinsic. It's a certain result we obtain after abiding by a set of guidelines and achieving a particular objective. An even better illustration of an intrinsic reward than money is the agent's enthusiasm to learn new skills that could come in handy later on.
Downloads
References
Ng, A. Y., & Russell, S. J. (2000). Algorithms for inverse reinforcement learning. Proceedings of the Seventeenth International Conference on Machine Learning, pp. 663–670.
Karpathy, & M. Van De Panne. (2012). Curriculum learning for motor skills.
Barto, A. G. (2013). Intrinsic motivation and reinforcement learning. Intrinsically Motivated Learning in Natural and Artificial Systems, Berlin, Heidelberg: Springer, pp. 17–47.
Wilson, A. Fern, & P. Tadepalli. (2014). Using trajectory data to improve bayesian optimization for reinforcement learning. J. Mach. Learn. Res.
N. Bougie, & R. Ichise. (2020). Skill-based curiosity for intrinsically motivated reinforcement learning. Mach. Learn.
T. D. Kulkarni, K. R. Narasimhan, A. Saeedi, & J. B. Tenenbaum. (2016). Hierarchical deep reinforcement learning: Integrating temporal abstraction and intrinsic motivation.
Abhay Singh Hyanki, Shweta Meena, & Tarun Kumar. (2021). A survey on intrinsically motivated reinforcement learning. International Journal of Engineering Research & Technology, 10(5), 1150-1153.
Kulkarni, Tejas D., Narasimhan, Karthik R., Saeedi, Ardavan, & Tenenbaum, Joshua B. (2016). Hierarchical deep reinforcement learning: integrating temporal abstraction and intrinsic motivation. Proceedings of the 30th International Conference on Neural Information Processing Systems.
R. Salakhutdinov, & A. Mnih. (2008). Bayesian probabilistic matrix factorization using markov chain Monte Carlo.
Raffin, S. Höfer, R. Jonschkowski, O. Brock, & F. Stulp. (2017). Unsupervised learning of state representations for multiple tasks. ICLR.
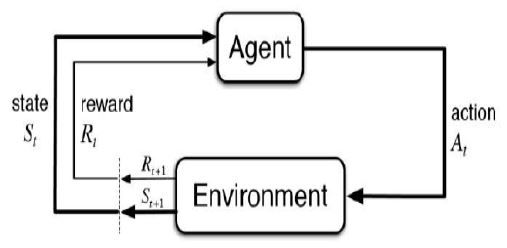
Downloads
Published
How to Cite
Issue
Section
ARK
License
Copyright (c) 2024 Laxmi Gautam, Rajneesh Kumar

This work is licensed under a Creative Commons Attribution 4.0 International License.
Research Articles in 'Applied Science and Biotechnology Journal for Advanced Research' are Open Access articles published under the Creative Commons CC BY License Creative Commons Attribution 4.0 International License http://creativecommons.org/licenses/by/4.0/. This license allows you to share – copy and redistribute the material in any medium or format. Adapt – remix, transform, and build upon the material for any purpose, even commercially.