A Study on Deep Learning Architectures and Dimensionality Reduction Techniques on Gene Expression Data
DOI:
https://doi.org/10.5281/zenodo.11211521Keywords:
biomarkers, cancer prediction, deep learning, dimensionality, epigenetics, feature learning, gene expressionAbstract
Genomics, driven by the evolution of high-throughput sequencing and microarray technologies, has become one of the key inventions of cracking the secrets of complex biological systems. The deep learning architecture not only provides with a powerful tool to derive the hidden insights from the huge amount of genomic data, but also enables to mine meaningful information. In this study, we will examine the application of deep learning methods in the analysis of genomics data, specifically on dimensionality reduction and predictive modeling for binary phenotypes. We focus on the problems with the existing strategies, spot the avenues for the further research, and provide you with a glimpse of the dramatic influence of deep learning on genomics. In this study, we delve into the application of deep learning methods in the analysis of genomic data, with a specific focus on two crucial aspects: dimensionality reduction and predictive modeling for binary phenotypes. Dimensionality reduction techniques are essential for tackling the high-dimensional nature of genomic data, where thousands or even millions of features (e.g., gene expressions, genetic variants) are measured for each sample. Deep learning models can effectively capture the complex relationships and patterns within this high-dimensional space, enabling the extraction of lower-dimensional representations that preserve the most salient information. Throughout this study, we critically examine the existing strategies and approaches in the field of genomics, identifying their limitations and highlighting the avenues for further research. We explore how deep learning can address these challenges and provide a glimpse into the dramatic influence this technology is poised to have on the field of genomics.
Downloads
References
S. R. Oshternian, S. Loipfinger, A. Bhattacharya, & R. S. N. Fehrmann. (2024). Exploring combinations of dimensionality reduction, transfer learning, and regularization methods for predicting binary phenotypes with transcriptomic data. BMC Bioinformatics.
Bo Chen, Wai Lam, Ivor W. Tsang, & Tak-Lam Wong. (2012). Discovering low-rank shared concept space for adapting text mining models. IEEE Transactions on Pattern Analysis and Machine Intelligence, 1284–1297.
Amel Ghouila. (2009). Application of Multi-SOM clustering approach to macrophage gene expression analysis. Infection, Genetics and Evolution, 328-336.
Saad Sahriar, Sanjida Akther, & Jannatul Mauya. (2024). Unlocking stroke prediction: Harnessing projection-based statistical feature extraction with ML algorithms. Heliyon.
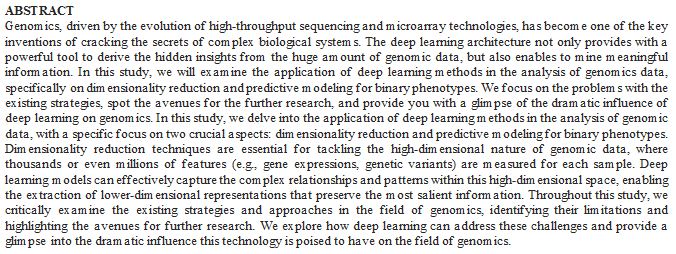
Downloads
Published
How to Cite
Issue
Section
License
Copyright (c) 2024 Remyamol K M

This work is licensed under a Creative Commons Attribution 4.0 International License.